Professional associations are reasserting AI guidance: adult supervision is required
Among the dramatic developments in machine learning in the last couple of years, the emergence of new and spectacularly capable large language models (LLMs) is particularly striking. Their sophistication makes it easy to be misled by the capabilities they offer, but failure to check the output can lead to disaster.
LLMs impress with style and fluency more than accuracy
Some commentators see such LLMs – of which ChatGPT is the poster child – as a step on the road to an artificial general intelligence (AGI). As their text interface is so flexible, and the scope and style of the responses they can provide is so wide, the leading-edge LLMs do indeed offer some of the capabilities of an AGI. They ‘look like a duck and quack like a duck’, at least from certain angles, so that point may be true. However, despite the shockingly smooth prose they offer, the actual quality (as in accuracy and coherence) of the underlying facts/opinions offered by these LLM systems remains poor, even if it is improving. They are not yet ‘superhuman intelligence’. The smoothness continues when they rather maddeningly apologise fluently for a mistake in their previous response when you point it out – but it can, and does, mask serious errors. Whether you call it ‘hallucination’ (or indeed ‘artificial inanity’), you cannot trust the LLM even if there are pearls amongst the swill, even – especially – if it is mostly pearls. Similar worries apply to the output of the text to image models such as DALL-E: they can look superficially plausible while containing impossibilities, such as a person riding a bicycle with no pedals.
In effect, the collective AI industry’s training processes have taught these models how to appeal to our ability to detect lack of fluency, without yet being able to train them to care about representing the truth. Put another way, instead of being a colleague who can help, an LLM is a ‘new intern’ without the benefits interns offer of not wanting to mislead you: LLMs are not sensitive to social pressure or incentives outside the training process, the prompt and its context. Interns will put to use their education and feedback from colleagues, and get better at the task, and in the end may become highly respected colleagues. Of course, analogous educational, feedback or incentive mechanisms could potentially offer means by which the future domain-specific LLM performance could yet be improved.
Professional regulators will issue guidance but sometimes this will be ignored
Given these dramatic developments, and given that many actors already want to use these LLMs in a wide variety of real-world applications, some kind of regulation is going to be considered. This may bear on many sectors, and cover many of the potential ills that AI may cause, as well as getting in the way of many of the benefits that these systems may be able to provide in, for example, automation or better optimisation. Such regulation is a much larger subject than can possibly be covered in one small article such as this. But one tractable and well-scoped subset of regulation is in encouraging professionals to operate in a professional way. In the last year, several professionals have done the wrong thing and used LLM output directly as if it were their professional opinion without checking every word. This has included e.g. lawyers whose pleadings included hallucinated cases, and in one instance an academic’s submission to a parliamentary enquiry citing a hallucinated link between an accounting firm and a scandal. Similar risks even apply in LLMs used in systems built for internal use and trained on internal datasets: plausible falsehoods in the results will cause loss of efficiency, not gains in efficiency.
So professional regulators (of lawyers, engineers, medics, accountants etc.) are making or reiterating guidance to their respective regulated professions: LLM output cannot be used verbatim for professional purposes unless the responsible professional has checked every word, which means more than just ‘skim reading’, and that anyone contravening this will be found to have been reckless and is likely to suffer sanctions. Despite this, we will undoubtedly see and hear of many more such cases. At the same time, less-regulated or unregulated professions such as software development, journalism and consulting that do not currently operate within a firm framework of professional regulation will have a similar need for integrity and high personal standards in e.g. checking their sources and testing their outputs. There may even be opportunities for the further development and support of professional regulation and standards in these industries (e.g. recent initiatives of the BCS and Alliance for Data Science Professionals) – but, as in other policy areas related to GenAI, this field is developing so quickly that it is necessary to make the best progress we can with what we already have.
Thus, adult supervision is definitely required (as in ‘apparently even adults will need to be supervised’).
About us
Analysys Mason provides wide-ranging policy and regulatory advice to clients in the public sector, IT, telecoms and postal industries. The scope of risks introduced by LLMs is much wider than many other computer systems, because there is a tendency to anthropomorphise and ascribe agency where there is none (or where this is severely lacking). We can help clients understand, assess and mitigate these risks, as well as navigate regulation; in some applications that is going to require human agency. For further information, please contact James Allen, Partner, and David Abecassis, Partner.
Author
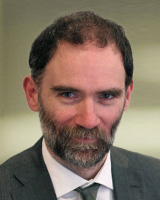