AI could transform RAN automation – if the ecosystem collaborates
16 January 2024 | Research
Caroline Gabriel | Adaora Okeleke
Article | PDF (3 pages) | AI and Data Platforms| Wireless Technologies
Automation of the radio access network (RAN), the most expensive element of a mobile communications service provider’s (CSP) asset base, plays an important role in cost-effective strategies aimed at reducing opex and improving the 5G business case. A new in-depth survey of 36 Tier-1 CSPs, conducted by Analysys Mason in 3Q 2023, revealed that 62% of CSPs aim to achieve automation of over 50% of RAN functions and processes by 2027. However, about two-thirds of CSPs have, so far, automated fewer than 20% of RAN tasks. Many are looking to AI to increase their automation levels and the intelligence of their RANs within the next 3–4 years, and our report provides details of how they aim to achieve that goal.
Analysys Mason’s new survey shows that CSPs are actively planning to adopt AI in RAN management
Among the CSPs surveyed, 31% aim to have automated 51–75% of their RAN processes by 2027, while 21% are targeting 76–85% automation. These targets are likely to prove to be over-ambitious in most cases. Previous RAN automation initiatives, often based on self-organising network (SON) technology, have consistently under-delivered compared to CSPs’ stated targets. However, many CSPs believe that AI will address the shortcomings of previous generations of RAN automation and are highly active in testing AI technology. The operators selected for our survey were required to be actively evaluating RAN AI, but 94% of them had already gone beyond that stage and were engaged in at least one test or trial. For some CSPs, this is the first attempt to automate the RAN – 25% of current RAN AI trials are by CSPs that currently manage their RANs entirely manually.
The CSPs are targeting a wide range of business benefits from RAN automation, enabled or enhanced by AI. Their targeted results fall into three broad categories – total cost of ownership (TCO) reduction, new services/revenue enablement and improved quality of experience. 89% of CSPs believe RAN AI will reduce TCO, and about two-thirds expect reduced downtime by having a more self-healing and predictive network, as well as improved spectrum efficiency.
To date, most CSPs are focusing on AI’s efficiency benefits, such as reduced TCO and time to market (TTM), rather than testing its ability to generate new revenue. Survey respondents indicate that they are less able to predict and quantify the impact of AI on new services, as opposed to resource efficiency. However, they also indicate that they will not make the transition from trials to full deployments unless they believe there are benefits that go beyond just efficiencies, such as improving quality of service (QoS).
Figure 1: Benefits being targeted for RAN AI investments1
CSPs are prioritising use cases that will enable the automated RAN to deliver beyond just efficiencies
CSPs’ ambition to leverage RAN AI for a wide variety of business outcomes contrasts with the narrow efficiency focus of previous automation initiatives such as self-organising network (SON). When the survey respondents were asked to share the use cases that they were evaluating for RAN AI support, they identified 17 categories. Only half were continuations of conventional SON automated tasks such as traffic steering, while the rest were either new, such as RAN slicing, or designed to transform tasks that are currently done manually, such as location awareness. Only 20% of these categories related to the radio unit alone, indicating the need to look beyond traditional RAN processes in order to maximise ROI in AI. CSPs are increasingly thinking about the application of AI in terms of the entire RAN system as they implement processes such as energy management, intelligent load balancing, dynamic spectrum usage and slicing, that require a holistic view of the RAN.
This highlights one clear difference between RAN AI strategies and the strategies adopted for SON. Centralised SON was challenging to deploy, especially in non-cloud environments, because of limited multi-vendor compatibility and fulfilment of high latency requirements. The extension of automation to network-wide use cases is one way in which RAN AI may address the shortcomings of SON in meeting the needs it was designed to address. This is driven by RAN AI’s potential capability to consume (dependent on access to available data) and automatically process large data volumes from multi-vendors quickly to derive relevant insights to support RAN automation.
There are challenges that the ecosystem must address before CSPs will deploy at scale
Despite the broadening scope of CSPs’ plans, there is still a gap between the theoretically perceived benefits of RAN AI and proven returns that would trigger a major investment. About half of the respondents in our survey said that new enterprise services revenue would be among the top three triggers for implementing full-scale RAN AI systems, but only one-third of respondents currently say they understand how these revenue streams would be enabled in the near term.
Before CSPs gain confidence in the upside of RAN AI, there are several important conditions that need to be in place. Many of these conditions relate to the maturity of RAN solutions and suppliers, contributing to effective risk mitigation. For instance, effective implementation of AI in the RAN will require Open APIs to ease data collection for training and deployment of AI models for inferencing.
Other important requirements include data frameworks (because existing frameworks are not suited to support real-time processing and decisioning requirements needed to drive RAN AI), sufficient cloud and RAN processing performance and acceleration to handle 5G and AI workloads optimally. Access to rich data sets and cost-effective skills and operations are also considered to be important as one-third of respondents identified these requirements among their top three challenges to address before deploying RAN AI at scale.
Most of these challenges will take some time for the ecosystem to address, but are essential to convince most CSPs of the comprehensive business case. Overall, 44% of CSPs expect RAN AI solutions to be mature and fully deployable only in 3–4 years from now, and 26% think it will take longer. Availability of affordable skills is believed to be the biggest challenge in terms of timing, as well as the wait for proven use cases and case studies, rich data sets and data frameworks.
It is essential that members of the RAN and AI sectors work closely together to address these challenges and unlock the potential of this emerging technology. Almost 60% of CSPs plan to rely on a combination of AI specialists and RAN vendors, rather than their traditional suppliers alone. If vendors from both sectors take a collaborative approach, this could help to shorten the timeframe for large-scale RAN AI deployment, and hasten the achievement of tangible impact on efficiency, quality of experience and revenue.
1 Question asked ‘What are the top three benefits you are targeting from implementing RAN AI in the coming 3–5 years?’; n = 36 executives from 36 Tier-1 mobile operators; 3Q 2023.
Article (PDF)
DownloadAuthors
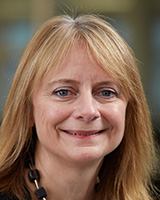
Caroline Gabriel
Partner, expert in network and cloud strategies and architecture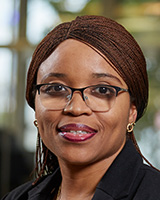