Generative AI presents opportunities as well as challenges for the telecoms industry
11 May 2023 | Research
Adaora Okeleke | Joseph Attwood
Article | PDF (3 pages) | Data, AI and Development Platforms
Communications service providers (CSPs) are beginning to explore the use of generative AI (GenAI) in telecoms.1 Early use cases are centred around improving customer experience. CSP vendor partners like Microsoft also plan to use this technology to enhance software development practices in telecoms. However, to support the introduction of GenAI, CSPs will need to develop more robust data architectures and implement more effective data governance.
GenAI can help CSPs to improve customer experience and fill software skill gaps
GenAI has the potential to address key issues that CSPs are facing with their digital transformation programmes. CSPs and vendor partners are already exploring the use of GenAI in the following use cases.
- Customer service operations. Korea Telecom (KT) has developed a GenAI tool, MI:DEUM, which will be used to perform call centre tasks such as responding to customer queries with improved intelligence. China Telecom is developing a ChatGPT-like service for industrial use, both internal use cases, for customer service functions and for supporting services targeted at its enterprise customers. Chatbots have played a critical role in performing these tasks to improve customer experience. However, chatbots are mostly restricted to helping customers perform basic tasks such as paying bills and making purchases, with limited ability to address more complex issues. Chatbots based on GenAI have a much broader range of potential use cases (based on the robust training processes used to create the language models), including supporting customer service agents with customer facing or back-office operations. However, it is a nascent technology and will need to be tested extensively before it is used to engage directly with customers.
- Unified customer experience. GenAI can be used to provide unified experiences to customers, across all services offered by CSPs. For example, SK Telecom’s soon to be launched A. (A dot) combines the conversational ability of models like ChatGPT with task-oriented dialogue when customers are using SK Telecom’s digital services. Through conversations with A., customers can access SK Telecom services including music streaming, e-commerce and digital payment services and perform actions such as send texts, make calls and manage their schedules.
- Software development. CSPs currently face a skills gap when it comes to software development skills. The adoption of GenAI could increase the productivity levels of network engineers with software expertise, allowing CSPs to gain more with less resources, thus fast tracking the pace at which they automate their networks. Microsoft is developing a telco-specific GenAI that can write code for network operations.
CSPs’ current data architectures and governance practices cannot support GenAI effectively
Despite these opportunities, there are clear challenges that would affect the pace of adoption of GenAI in telecoms.
GenAI models require very large data sets (billions of data points), to train them, with data sources spanning numerous locations, types and formats. OpenAI’s GPT-3 model used to create ChatGPT, for example, was trained using 45TB of text data obtained from several sources across the internet.
Unfortunately, access to and the management of high-quality data remains the bane of AI projects within telecoms. Data access is limited for several reasons including systems having proprietary interfaces and data pipelines failing due to code bugs, badly formatted data or poorly configured servers for monitoring. Text-based data sources, such as vendor operations manuals, will need to be accessed to train GenAI models. Processes involved in using these types of data can be challenging, expensive and can take time. More importantly, CSPs use several network equipment vendors. Managing and aligning data from these providers for GenAI will be challenging. These issues could affect the accuracy of results gained from GenAI models.
In addition, CSPs need better governance of data and AI to ensure ethical concerns such as ensuring customers’ data privacy is protected and effectively addressing bias in the content provided by GenAI. The increase in volumes and distribution of data required to implement GenAI make it difficult for CSPs to implement and scale data and AI governance practices.
Adopting GenAIdemands robust data architectures and data governance
The opportunities and challenges surrounding GenAI in telecoms call for CSPs and industry stakeholders to take actions that facilitate the effective deployment of the technology. This includes transforming existing data architectures, improving data governance practices and exploring the role of the public cloud in supporting GenAI in telecoms.
CSPs need a data architecture that can deal with the massive data workloads that will be required for the training and lifecycle management of GenAI models. Data platforms used by CSPs should be robust and flexible and support the requirements given in Figure 1.
Figure 1: Requirements of data platforms used to support GenAI
CSPs will need to improve data governance practices. This will require all industry stakeholders including CSPs, application vendor partners, public cloud providers, professional services providers and industry bodies, to work closely to define and implement the required guardrails required to avoid threats such as the infringement of customers’ data privacy from occurring. Industry stakeholders will also need to decide the levels of transparency that GenAI model developers need to fulfil to ensure the traceability of these models.
In order to support GenAI, it is crucial to identify the deployment environment for data. Given the data storage capacity and cost to set up the data platforms required for the development of these models, we expect CSPs to defer to public cloud data platform services to support these requirements. Microsoft’s activities in this domain potentially puts them in a favourable position. Regulatory policies around data sovereignty will, however, require some data sets to be on-premises. Therefore, hybrid data architectures will be needed to connect data on-premises and in the cloud.
GenAI is a nascent technology not just to telecoms but other industries. However, if the industry is to capture new opportunities with this technology, then early discussions to facilitate adoption will be critical.
1 Generative AI refers to a category of artificial intelligence (AI) algorithms that generate new outputs based on the data they have been trained on. ChatGPT is a use case of GenAI.
Article (PDF)
DownloadAuthor
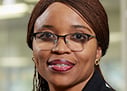
Adaora Okeleke
Principal Analyst, expert in AI and data management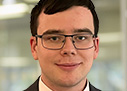
Joseph Attwood
AnalystRelated items
Strategy report
Retrieval-augmented generation: considerations for GenAI platform vendors targeting telecoms operators
Article
Telecoms operators’ approaches to GenAI solutions should be shaped by priorities, experience and budget
Tracker
Public cloud provider and CSP partnership tracker 1H 2024