Understanding the cost of quality of service
Many operational issues affect quality of service due to the conflict between time-varying demand and a finite quantity of resources. A classical telecoms example of this balance is illustrated by the probability that you can get a connection when you dial another user during the busy hour. Operators must consider a similar situation when providing field force to respond to network faults; here, a service level agreement (SLA) such as “fix a fault by the end of the next working day after the fault report” will be met some percentage of the time over the year (for example, 85%), and this percentage will depend on the number of technicians available to fix faults. There are plenty of other examples that affect each of us every day, including the time we spend in queues in shops and the response times of web servers.
Models are needed to understand the impact of increasing the quantity of resources on whichever statistic is of interest (such as the probability of meeting the SLA). In the case of voice calls, the probability of a successful connection depends on the mean demand, the average call duration and the number of channels, and is given by a relatively simple formula. Erlang worked out the mathematics for this in 1910. This formula helps us to see how having aggregated demand and a larger pool of resources leads to improved performance (the SLA will be met a greater proportion of the time). By analogy, considering the response to faults scenario above: if travel time were not an issue, we would be encouraged to treat the field force as a single, larger pool of resources, rather than several local teams. One additional notable point is that the formula is not easily inverted; we can easily calculate the performance of a specified set of resources, but we cannot directly determine the amount of resources needed to reach a given performance level. This means that it is more practical to calculate the performance of many different resource levels and interpolate.
Figure 1: Illustration of the probability of the delay being less than a specific target level as a function of the number of servers, using a standard random arrivals, negative exponential service time queueing model (‘M/M/c’) (with the mean arrival rate λ=49.9 per day and the average service rate μ=0.5001 per day)
Source: Analysys Mason, 2019
If we change the assumptions, for example if the job arrivals (such as faults occurring or customers dialling) are no longer random, or if some jobs take priority over others, then even though some of these cases have known solutions that can be calculated efficiently we may need to move beyond these analytic methods and use simulations instead. Simulation allows us to go beyond the simplified cases: for example, we can add prioritisation, multiple classes of job, different types of resources, time-dependent demand, situations in which the first service attempt fails, and the effects of the working week. Such models are powerful, though they are harder to build than the analytic models (although modern tools can make this much easier). They are also harder to audit, and harder to use to generate understanding. Rare events can be particularly difficult to study, simply because they do not often occur. The volumes of data involved can be large, which makes it challenging to see patterns. Long runtimes are possible and can impede usability, even with well-chosen tools, modern computer hardware and many copies running in parallel. Specialists are required in such instances.
Analysys Mason recently performed two projects for Ofcom, looking at how the number of field force staff affected the repair and installation quality of service. In one case, we assisted Ofcom in significantly increasing the capabilities of its own model; in the other, we audited Openreach’s internal model. Both models were simulations built in Python, with significant similarities, albeit taking different approaches and making different simplifying assumptions. Both could use real world data about demand. Ofcom used the results of these projects to support its estimates of the cost implications of significantly increasing the required target quality of service levels for fault repair and installations within Openreach's UK local loop network.
If you would like more information about understanding the relationship between resource levels and the quality of service perceived by end users, please contact James Allen.
Downloads
Article (PDF)Authors
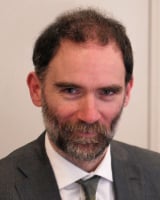