FutureNet World 2024: operators are progressively adopting AI technologies but still face issues with scale
07 May 2024 | Research
Joseph Attwood | Adaora Okeleke
Article | PDF (3 pages) | Data, AI and Development Platforms
Analysys Mason attended FutureNet World 2024 in London, UK in April 2024. Operators’ use of AI to support their network automation strategies was a key focus of the conference. Attendees had a far more concrete understanding of generative AI (GenAI) than at last year’s event, but they also continued to stress the importance of non-generative AI (non-GenAI) technologies for network operations.1 Operators acknowledged that they will eed to provide the right tools to developers and data scientists, rethink their team structures and improve their data foundations in order to scale their AI deployments. This article presents Analysys Mason’s main takeaways from the event.
Operators should not let the hype distract them from addressing their concerns with GenAI technology
Deutsche Telekom’s (DT’s) Vice President of Technology Strategy, Ahmed Hafez, was forthcoming during a presentation at the event about the operator’s use of GenAI to improve employee productivity. Hafez said that DT had numerous GenAI-based use cases in production, including a coding assistant for developing test cases and an “FTTH roll-out chatbot” that helps contractors to adhere to regulation during fibre broadband installation. This chatbot uses retrieval augmented generation (RAG) based on information gathered from around 400 documents, each of which has about 900 pages, to respond to contractors’ questions and provide links to the relevant sections within these documents.
However, operators remain apprehensive about exposing GenAI capabilities to customers. Jaime Tatis, TELUS’s Chief Insights Officer, said, during a panel discussion, that the operator has been slow to implement external-facing GenAI use cases due to the need for extensive adversarial testing. Orange said that it was especially cautious of implementing use cases such as customer-facing GenAI chatbots due to the regulatory and liability issues caused by inaccurate responses.
Other panellists indicated that they continue to face difficulties when integrating GenAI models with their backend systems to automate business processes and operations.2 Operators stand to make productivity gains from the knowledge mining and content generation capabilities of GenAI, but they will derive the most value from automation-driven use cases. Operators must therefore address their concerns about using GenAI in these use cases urgently.
Non-GenAI still plays a critical role in driving operators’ network automation strategies
Operators at FutureNet World 2024 reported that they are still investing in non-GenAI tools and are scaling their use of this technology in use cases such as anomaly detection, predictive maintenance and smart planning, following positive early results. For example, Three UK’s Iain Milligan revealed that the operator had achieved energy savings of 7–10% by employing Ericsson’s AI-driven intelligent power-saver solutions. Ahmed Hafez explained that DT is primarily focused on using non-GenAI for network-related AI activities (70% of efforts for these activities use non-GenAI, compared to 30% for GenAI). Operators should consider how to enable non-GenAI technologies to work alongside their GenAI counterparts, perhaps by using insights from non-GenAI models as key prompt components for GenAI models.
An appropriate balance between centralising AI use case development practices and disaggregating them is needed to accelerate AI adoption
AI adoption will scale effectively when operators have a skilled and well-equipped AI organisation; achieving the optimal organisational structure will be key to success. Operators including Liberty Global and DT shared that their AI projects are generally centrally managed at the group level; a centralised team provides best practices, assets, tools, reference architecture and governance frameworks. However, the actual implementation of AI use cases is normally the responsibility of decentralised teams due to the unique requirements of each operating company within the group and the differences in data available to each team. DT explained that it encourages decentralised development, even at the expense of some duplication of functions, because this results in far faster implementation than centralising all development activities.
Such experiences suggest that operators should encourage development across multiple teams and opcos, and should establish centres of excellence3 to share and collate best practices for AI implementation.
Furthermore, operators should attempt to democratise the creation of AI tools to maximise the value of AI technology. Hafez explained that DT is aiming to enable over 80% of its network experts to create and manage AI applications, but this requires that these experts have the required skills and toolsets. Similarly, TELUS has created an internal self-service platform that allows employees to experiment with, and develop their own, AI use cases.
Operators agree that tackling data challenges will be critical to deploying cost-effective AI solutions
Operators need to rethink their data strategies to tackle issues such as data quality, the increasing number of data sources, data replication and data governance before they can scale their AI developments. Figure 1 provides a summary of the solutions mentioned by operators at FutureNet World 2024 that can address current data challenges.
Figure 1: Overview of how operators can address data challenges
Telefónica’s Carlos Medina Gonzalez indicated that operators should develop a data management framework when implementing these solutions. They should have defined roles and responsibilities for staff and should invest in technology that meets the requirements of clearly defined use cases. A data operations (DataOps) team will be critical to ensure that processes that are designed to drive continuous access to high-quality data assets are maintained regularly.
Our research also indicates that operators that wish to fast track their AI-related projects require truly desiloed data architectures that run in hybrid cloud environments, integrate directly with data sources and can be updated to align with the evolving data needs of AI technologies.
1 Non-GenAI technologies are AI-related technologies, such as predictive AI, that can analyse large volumes of data to make predictions, decisions and/or classifications. They are developed using smaller models than those used for GenAI and address specific tasks.
2 Analysys Mason also identified the challenge of integrating GenAI models with internal systems in Market insights: a guide for CSPs on maximizing the value of GenAI.
3 For more information about the experiences of early adopters of GenAI, see Analysys Mason’s Building the operator business case for GenAI adoption.
Article (PDF)
DownloadAuthors
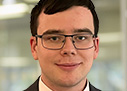
Joseph Attwood
Analyst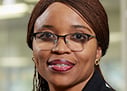
Adaora Okeleke
Principal Analyst, expert in AI and data managementRelated items
The Microsoft–Mistral AI partnership will bring both benefits and challenges for telecoms operators
Article
GenAI and the telecoms sector: three GenAI platform implementation strategies
Strategy report
Data, AI/analytics and development tools M&A tracker 2H 2023