AI could be a wasted investment without good data and processes
Preparing the ground in advance gives AI the best chance of success
As companies rush to embrace AI and to understand how it can enhance their businesses, they are likely to realise that AI is not the silver bullet they had hoped for. Having the right skills, knowledge of data and ability to understand the business end to end is a prerequisite. Many companies do not have these skills in house, and so finding the right partners can be a critical aspect to turn AI’s potential into a concrete competitive advantage. Ultimately, the greatest benefit from integrating AI will go to those organisations that have clear, organised business processes and data.
Current AI use cases are fundamentally about delegating the tasks of searching, analysing, presenting and predicting data, or making autonomous decisions via statistical algorithms with the aim of gaining effectiveness and efficiency on a scale that has not been possible previously. This is true for automating processes, optimising digital channels for pricing and enhancing customer experience. But as delegation comes with an element of reduced control, it is crucial that the quality of the outputs provided can be assured as much as possible.
Clarity of purpose is vital
A critical ingredient for success with AI relates to clarity of objective: organisations must be clear on the role they want AI to play and the questions that they are asking AI to solve, or the type of processes that they want to automate.
Once clear objectives are set, success is highly contingent on data: if the right data is not available (in terms of quality, quantity, accessibility and completeness), companies will face a significant challenge simply getting to the starting point.
Large, mature companies typically hold years (or even decades) of data on their business operations and their customers, but often this data is dispersed across several legacy systems, contains duplicates and errors, and is not easily accessible in real time.
Expecting any form of AI tool to operate effectively and independently in this sort of data landscape will cause significant problems. In addition to the well-publicised hallucinations from large language models (LLMs), it is increasingly likely that price recommendations or transaction summaries presented to customer service agents will be incorrect, since they are based on inaccurate or incomplete data. AI processes can be opaque, meaning that, if decision processes are largely delegated to AI, the output will be difficult to validate.
Data quality dictates the value of AI tools
Many companies do not have easy access to all the data they would want, and so are running their businesses using proxy measures. They may have multiple versions of the same measure reported in different ways in different places, based on different business scenarios and different timescales. AI itself will not understand why an organisation has several measures and definitions of customer churn, for example.
As companies have evolved over longer periods of time, they will have been shaped by both their technical capability and the market around them. Business processes and controls will have evolved in the same way. In this environment, formulating a concrete, tangible and solvable task for an AI tool might become more complex than implementing the AI solution itself.
Then there is little point in developing the ability to find that solution in record time with the goal of identifying changes or recommending actions without the ability to approve the recommendation and to implement it quickly. For this reason, a truly holistic view of the business is required to understand not only the impact of AI on governance, decision-making and controls, but also the actual technical ability to use the outputs from AI in a timely manner, whether that be for dynamic price adjustments or real-time marketing decisions.
Younger, simpler, and smaller companies are most likely to be in a better starting position here, and the fact that they have simpler processes and governance will make them better positioned to exploit the benefits of AI than their larger competitors.
Being well positioned to integrate AI is therefore much more complex than simply identifying a selection of use cases. It means having reliable, consistent, clean data on the company’s operations. It means having clear, well documented processes to offer a baseline against which to measure impact and success. It means having clarity on who is responsible for a particular process or decision, in order to agree on the intended benefit or the operational question or challenge being addressed. It also means aligning organisational and technical capabilities to ensure that AI-driven optimisation is unimpeded throughout the entire organisational environment.
Implementing AI solutions successfully requires companies to first understand and then address all of these issues. In a world of legacy systems and outsourced service agreements, many companies will find that they no longer have this capability in house, and so the first step to successfully implementing AI may well be finding the right partner: one that understands the abilities and boundaries of AI, and can apply this to the specific business context.
Working with an AI specialist must be a partnership
An AI specialist partner can be invaluable, but it will still fall to individual companies to provide the right level of business understanding. It is critical that companies are able to translate the detailed realities and constraints of their business in the right way, with dedicated and informed staff acting as that interface. This allows a clear, holistic view of the end-to-end business processes, business model and data, while at the same time understanding the art of the possible from different technologies.
About us
Analysys Mason has extensive experience in helping organisations across industries understand, analyse and migrate data in legacy systems. We also support clients in assessing and optimising business processes, and defining their future operating model, often acting on behalf of organisations as the bridge between their operation and the capabilities of new technology. For more information, please contact Stefan Hainz (Partner) and Paul Jevons (Director).
Authors
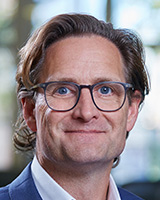
Paul Jevons
Director, expert in tech-enabled transformation